Value of Information Analysis
Section Editor: Koen Degeling, PhD
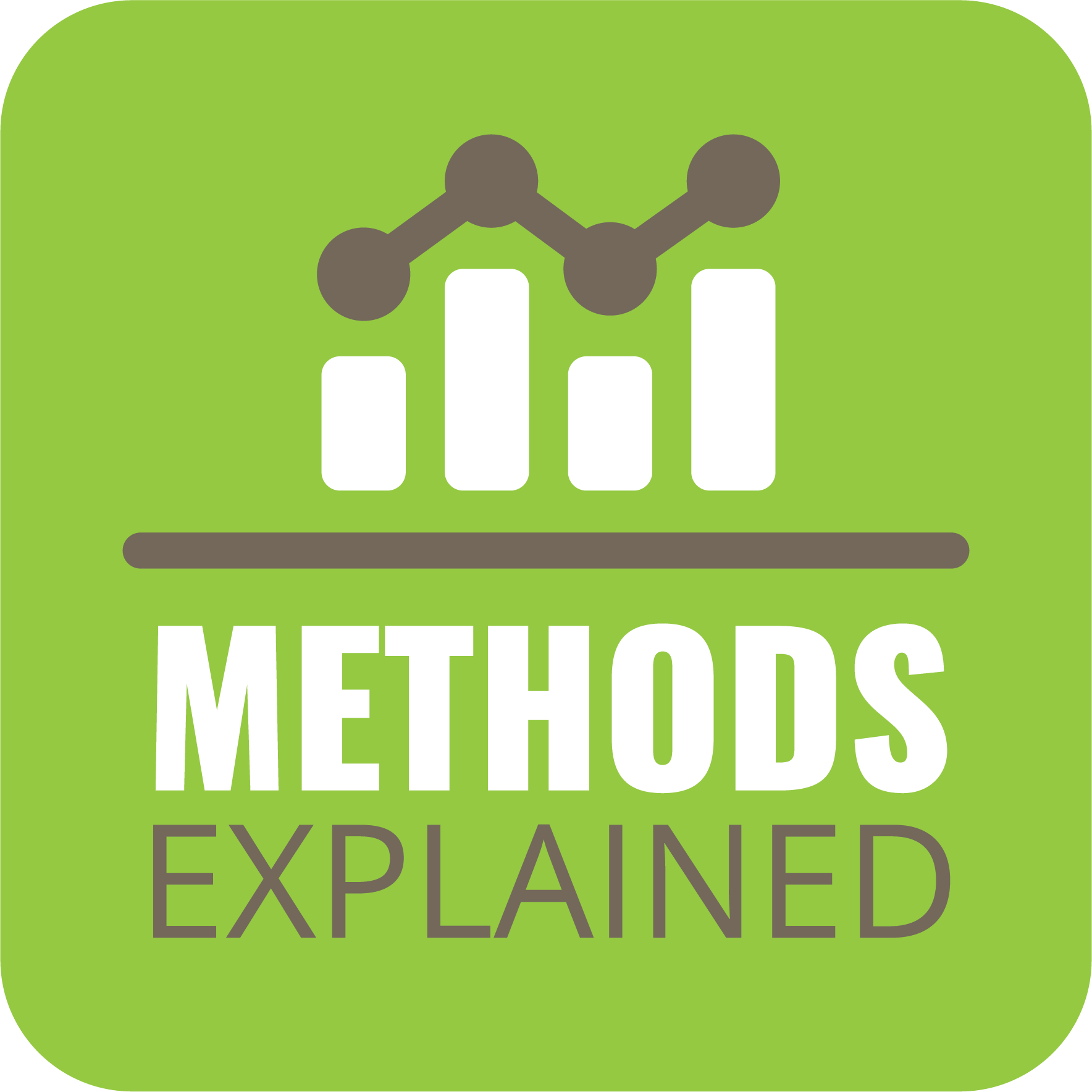
In this edition of Methods Explained we are covering value of information analysis (VOI) based on a conversation with 2 international experts on the topic, Saskia Knies and Natalia Kunst. Saskia Knies, PhD, is coordinating advisor at the National Health Care Institute in The Netherlands (Zorginstituut Nederland), which is the first health technology assessment agency in the world to mandate VOI in its guidelines, and a member of the ISPOR Task Force on VOI. Natalia Kunst, PhD, is senior research fellow (associate professor) at the Centre for Health Economics of the University of York, co-founder of the Collaborative Network for VOI, and author and editor of several publications on VOI.
What is the objective of VOI and what can it be used for?
VOI comprises a toolbox of analyses and metrics that can be used to provide a range of insights. Their aim is to achieve improved health outcomes at the same or lower costs through improving resource allocation decisions, eg, whether a new healthcare intervention should be reimbursed or not. These analyses quantify the uncertainty in such decisions and provide an understanding of the consequences of making a particular decision given the information at hand and of the value of performing further research before making the decision. Within the field of health economics and outcomes research (HEOR), VOI is typically performed using decision-analytic models as part of health technology assessments, such as cost-effectiveness models or other types of health economic models.
Given the uncertainty in the model parameters that arises from the evidence used, VOI determines the probability that the best decision according to the model turns out to be suboptimal when more evidence would be available, and estimates what the consequences are of making the wrong decision. A consequence can be that patients receive a suboptimal treatment, which can either be a less effective treatment or a cost-ineffective treatment.
These insights can be used as a type of sensitivity or uncertainty analysis to improve the understanding of a decision-analytic model by providing information on the drivers of uncertainty and whether those uncertainties have an impact on the decision. Ultimately, insights from VOI are used for research prioritization, ranking the value of proposed studies, and informing stop–go decisions. They can also inform the optimal design of future research studies.
In this issue of Methods Explained, we focus on the 2 most used VOI metrics: (1) the expected value of perfect information (EVPI), and (2) the expected value of perfect parameter information (EVPPI, also known as expected value of partially perfect information). The EVPI and EVPPI provide insight into the total amount of uncertainty and how that impacts the decision, and which parameters contribute most to this uncertainty.
On a high level, how does VOI work?
Although VOI is mostly applied in model-based health economic analyses, it can theoretically also be applied in data-driven studies, such as trial-based cost-effectiveness analyses. VOI takes the uncertainty from evidence used to populate the decision-analytic model and analyzes what the probability is that the wrong decision is made based on the model outcomes, and what the consequences of making the wrong decision are. These consequences are typically quantified in terms of health or monetary outcomes, such as the net health benefit or net monetary benefit. These outcomes are then extrapolated to the relevant (patient) population over a certain time horizon. The decision maker must interpret those findings and determine whether they are comfortable making the decision based on the current evidence or whether further research needs to be performed. This can assist in making the trade-off between making the decision with (potentially high) uncertainty or reducing this uncertainty through further research, as well as the resources required to do so.
For example, based on a given willingness-to-pay threshold per quality-adjusted life year gained, the EVPI may be $2 million for a given patient population over a 5-year period. This suggests that the decision maker may choose to delay the decision if they believe that all the uncertainty in the decision can be negated through further research that costs less than $2 million. However, it is typically not feasible to conduct research that collects enough evidence to negate the uncertainty in all model parameters. Rather, a particular research study would reduce the uncertainty in only specific parameters. To that end, an EVPPI analysis can be used to investigate which parameter or group of parameters contributes most to the uncertainty in the decision. In the example, it could be that an EVPPI of $1.5 million is found for the parameters on overall survival of the intervention and comparator treatments. In other words, the value of reducing the decision uncertainty by collecting perfect information on the overall survival parameters is $1.5 million.
If it is considered feasible to negate this uncertainty in the decision for that budget, additional VOI analyses should be performed to determine whether further research is indeed worthwhile and what the optimal study design is. This can be done by estimating the expected value of sample information and the expected net benefit of sampling.
What makes VOI different from other types of sensitivity and uncertainty analyses?
VOI is a decision-analytic method that can be described as an uncertainty analysis. While there are other sensitivity and uncertainty analyses that are well-known and often conducted as part of an economic analysis, such as one-way sensitivity analysis and probabilistic analysis (also referred to as probabilistic sensitivity analysis), there is a notable difference between these methods. A one-way sensitivity analysis provides insights into the impact of uncertainty in specific parameter(s) on the model outcomes. Probabilistic analysis is an uncertainty analysis that helps propagate uncertainty from the model input parameters to the outcomes of the model. The results of a probabilistic analysis can be used to assess the probability of cost-effectiveness for the decision options considered. VOI gives insight into uncertainty in the decision and the impact of specific parameters on this decision uncertainty. This is a fundamental difference, because even if outcomes are highly uncertain, there may be no uncertainty in the decision if the complete range of outcomes suggest one decision alternative is optimal (or not).
The difference between uncertainty in outcomes and decision uncertainty can be illustrated using the incremental cost-effectiveness planes presented in Figure 1. This figure shows 2 scenarios for a hypothetical cost-effectiveness analysis of a novel intervention Treatment A compared to Treatment B. Both scenarios presented in Figure 1 have the same amount of uncertainty in the outcomes, as demonstrated by the spread of the probabilistic analysis iterations (gray points) and corresponding confidence interval (black ellipse). There is substantial decision uncertainty in the left plot (Figure 1A) because the point estimate (black dot) suggests that Treatment A is not cost-effective and many iterations (gray points) are located above the willingness-to-pay threshold, but a substantial number of iterations suggest it may actually be cost-effective (a meaningful number of gray points are below the willingness-to-pay threshold). In the right plot (Figure 1B), however, there is no decision uncertainty because all iterations are located above the willingness-to-pay threshold, indicating that intervention Treatment A is not cost-effective.
This example illustrates that a probabilistic analysis only gives insights into the uncertainty around the outcomes and the probability of being cost-effective, whereas VOI quantifies the decision uncertainty and, therefore, is an important extension. Additionally, sensitivity analyses and probabilistic analyses do not give insights into the consequences of making the wrong decision, whereas VOI does quantify this impact on the net outcomes.
Figure 1. Incremental cost-effectiveness planes illustrating how the same amount of uncertainty in the outcomes can result in (A) substantial decision uncertainty and (B) no decision uncertainty, where the gray points represent the iterations of the probabilistic analysis, the black dot the mean outcomes, the black line the 95% confidence interval around that estimate, and the red dashed line the willingness to pay per quality-adjusted life year gained.
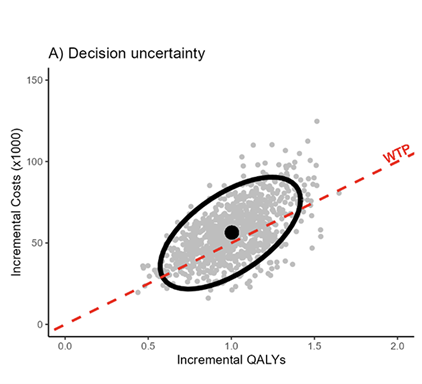
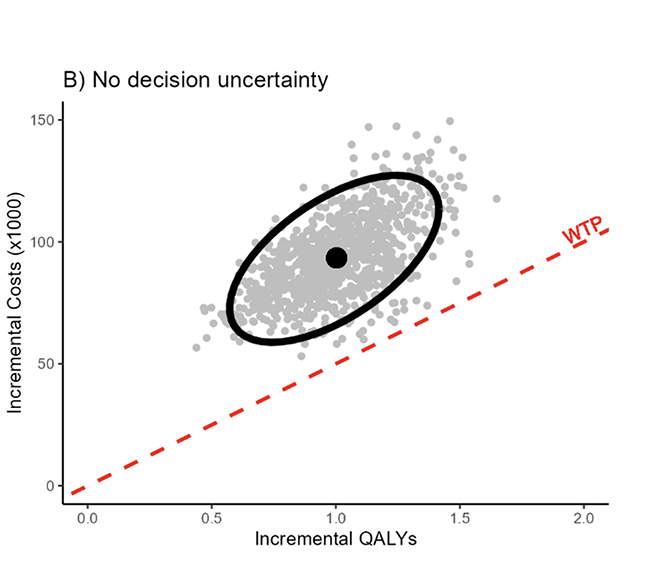
What steps are involved in performing VOI?
The reports of the ISPOR Task Force on VOI give an excellent overview of the steps involved with performing the different VOI analyses.1,2 In summary, a prerequisite to perform VOI is a clearly defined decision problem and a health economic model that is considered appropriate to inform decision making. A probabilistic analysis needs to be performed, including the assignment of appropriate probability distributions that describe the uncertainty in the model parameter values. The EVPI per patient can be calculated directly from the output of a probabilistic analysis and, hence, does not require additional analyses than typically performed. The EVPI per patient should be extrapolated to the total patient population over a specific time horizon.
The EVPPI can be obtained through a double-loop Monte Carlo simulation. This is comparable to performing a probabilistic analysis a few hundred or a thousand times, which can be computationally demanding and is not always feasible. Therefore, several approximation techniques have been developed to estimate the EVPPI based on the output of a standard probabilistic analysis. An example of this is the Sheffield Accelerated Value of Information tool.3
To what extent have these methods been used in practice?
Performing probabilistic analysis has become standard practice due to increased awareness and increases in computational power. As a logical extension of probabilistic analysis and due to the availability of guidance and increased awareness, the uptake of VOI has been increasing. Although initial applications have mostly been academic, a wealth of practical applications can nowadays be found in literature.
A key facilitator for the uptake of methods within the field of HEOR is inclusion in health economic evaluation guidelines. Therefore, an important milestone in the adoption of VOI is the inclusion of the EVPI and EVPPI as mandatory analyses in the 2024 update to the guidelines of the National Health Care Institute in The Netherlands.4 With this, the number of VOI analyses and consideration thereof in reimbursement decisions is expected to increase.
What are the remaining challenges in the adoption of VOI?
There are clear guidelines and practical examples that explain how VOI can be performed, although the HEOR community may not yet be familiar with the methods, what they can do, and how valuable the results can be.
An important challenge in the adoption of VOI likely is the interpretation of the results, as it may not be possible to clearly define what EVPI or EVPPI values are acceptable or when a decision may be delayed. Decision makers need to consider the results of VOI in the totality of information that is available to them, but this may be considered somewhat subjective in a context where consequences are high.
A frequently asked question is whether it is worthwhile to perform VOI if there is little uncertainty in the outcomes of the health economic analysis. The perception that performing VOI is only relevant when there is a large amount of uncertainty may also hamper the uptake. However, this is not necessarily true. There can be relatively little uncertainty in the outcomes, but the potential impact of that uncertainty on the decision can be high. The opposite can be true as well.
An important barrier to the use of VOI in practice may also be the disconnect between the funders of most research and the funders of healthcare. Currently, even when the funders of healthcare decide that the underlying evidence is too weak to reimburse a specific intervention, they may not be able to mandate further research to be performed by others.
What are some key references for further reading?
The reports of the ISPOR Task Force on VOI are a great starting point for those interested in learning more about the VOI toolbox and how they may use it in their research.1,2 Also, a book on VOI has recently been published.5 For a practical example of VOI, the study by Natalia Kunst et al on the clinical effectiveness and cost-effectiveness of population-based newborn screening for germline TP53 variants is an interesting read.6
References
- Fenwick E, Steuten L, Knies S, et al. Value of information analysis for research decisions—an introduction: report 1 of the ISPOR Value of Information Analysis Emerging Good Practices Task Force. Value Health. 2020;23(2):139-150.
- Rothery C, Strong M, Koffijberg H, et al. Value of information analytical methods: report 2 of the ISPOR Value of Information Analysis Emerging Good Practices Task Force. Value Health. 2020;23(3):277-286.
- Strong M, Oakley JE, Brennan A. Estimating multiparameter partial expected value of perfect information from a probabilistic sensitivity analysis sample: a nonparametric regression approach. Med Decis Making. 2014;34(3):311-326.
- Guideline for economic evaluations in healthcare (2024 version). National Health Care Institute. https://english.zorginstituutnederland.nl/about-us/publications/reports/2024/01/16/guideline-for-economic-evaluations-in-healthcare. Published January 16, 2024. Accessed November 11, 2024.
- Value of Information for Healthcare Decision-Making. Heath A, Kunst N, Jackson C, eds. Boca Raton, FL: CRC Press; 2024.
- Kunst N, Stout NK, O’Brien G, et al. Population-based newborn screening for germline TP53 variants: clinical benefits, cost-effectiveness, and value of further research. J Natl Cancer Inst. 2022;114(5):722-731.
We welcome your feedback on this article and any suggestions for methods to be covered in future editions. Send your comments and suggestions to the Value & Outcomes Spotlight Editorial Office.