Seeing the Bigger Picture: How Capacity Constraints Impact Value
Tara Li, PhD; Vladislav Berdunov, PhD, Putnam, London, England, United Kingdom; Christian Bührer, PhD; Oliver Cox, MRes, F. Hoffmann-La Roche Ltd, Basel, Switzerland; Roelien Postema, PharmD, Putnum, London, England, United Kingdom; Marloes Bagijn, PhD, F. Hoffmann-La Roche Ltd, Basel, Switzerland
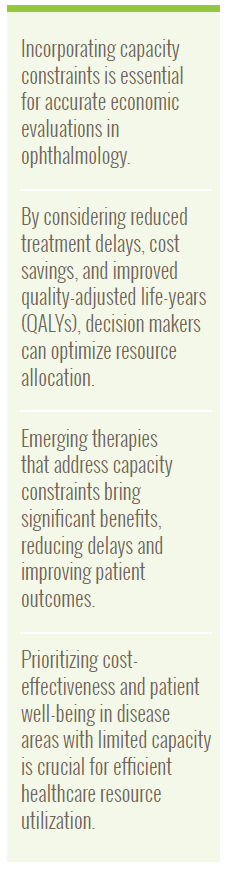
Introduction
Cost-effectiveness analysis is a key component of health technology assessment, which evaluates whether a new treatment provides value relative to existing health technologies available to patients. This analysis typically involves estimating the downstream effects of introducing the new treatment on healthcare costs and patient outcomes. Traditional cost-effectiveness analysis implicitly assumes that there is unlimited capacity in the healthcare system and does not consider capacity constraints that may impede or limit the implementation of treatments. In many chronic disease areas, including ophthalmology disorders, implementing effective treatment can be capacity-intensive because it often requires frequent specialized administration or monitoring. As a result, the timely provision of these treatments is capacity-dependent. In the presence of capacity constraints, treatment delays may lead to worsened health outcomes for patients.1
New treatments that alleviate capacity constraints would not be adequately valued if the positive impact on health outcomes of avoiding treatment delays is ignored, given that traditional cost-effectiveness frameworks do not explicitly consider the impact of capacity. Considering these capacity constraints and their impact on patients and healthcare systems when assessing the cost-effectiveness of new treatments in these disease areas may therefore be important to gauge an accurate assessment of relative value. This article explores the impact and importance of adding capacity constraints to cost-effectiveness analysis, illustrated using a case study of a simulation model for 2 treatments for neovascular age-related macular degeneration (nAMD) and diabetic macular oedema (DMO).
"In the presence of capacity constraints, treatment delays may lead to worsened health outcomes for patients."
Healthcare capacity constraints in ophthalmology
Most healthcare systems have to operate with constrained capacity, which includes limited availability of hospital beds, doctors, nurses, medicines, and other resources required to deliver care to patients. Ophthalmology is one of the areas that is most affected by capacity constraints due to the increasing prevalence of retinal conditions, aging population, and the uptake of resource-intensive treatments that require frequent administration as often as once every 4 weeks.2
Capacity constraints are a worldwide issue. As of September 2022, nearly 10% of National Health Service (NHS) waiting lists were in ophthalmology, with 657,222 people in England waiting for their first NHS ophthalmology appointments.3 Treatment delays caused by capacity constraints can have a significant impact on health; it is estimated that every month, approximately 22 people in the United Kingdom suffer from severe or permanent sight loss due to treatment delays in NHS eye clinics.4 While the Royal College of Ophthalmologists set a 2-week referral target,5 nearly four-fifths of optometrists report delays of 12 months or more for secondary care referrals, follow-up appointments, or treatments for their patients.1 Recognizing the exceptional strain on NHS capacity, the National Institute for Health and Care Excellence (NICE) published productivity objectives that include more durable therapies in ophthalmology that allow fewer interventions and appointments.6
The issue of capacity constraints is not unique to the United Kingdom. A survey of 49 retina specialists in Spain concluded that proper implementation of anti-VEGF treatment regimens is complicated by healthcare resource limitations.7 In France, an audit of intravitreal injection management in one ophthalmology department revealed a large increase in demand for intravitreal injections.7 Internationally, there is a lack of ophthalmologists to deliver eye care services.8
"Most healthcare systems have to operate with constrained capacity, which includes limited availability of hospital beds, doctors, nurses, medicines, and other resources required to deliver care to patients."
Why consider health system capacity in economic evaluation?
The emergence of sight-saving therapies with more durable outcomes and associated with more efficient use of resources (ie, longer treatment-free intervals between injections) offers an alternative. However, the traditional decision-making framework using cost-effectiveness analysis is not able to capture the value of reducing the strain on ophthalmology clinics, leading to undervaluation of treatments that can alleviate health system burden. This is illustrated using the conceptual example in the Figure. Economic evaluations that are able to quantify capacity constraints and incorporate them into cost-effectiveness analyses are needed in order to capture the true costs and benefits of treatments in disease areas where capacity constraints are an important consideration for healthcare decision making.
Figure. Conceptual example of capacity constraints in economic evaluation. The number of appointments required with a less durable treatment is represented by the blue line, and the number of appointments with the more durable treatment by the orange line. When considering the reality of overburdened health systems, the number of patients who can be treated in any given year is constrained, and any who cannot be treated immediately are placed on a waiting list. As such, the value of a more durable treatment that reduces the demand for appointments includes health system cost savings (solid shaded area), as well as avoidance of quality-of-life losses and costs associated with sight loss due to treatment delays (the pattern shaded area).
Ophthalmology case study to include capacity constraints in economic evaluation
To illustrate what impact capacity constraints could have on the cost-effectiveness of treatments for retinal conditions in the United Kingdom, a patient-level simulation model was developed.9 The model simulated a cohort of patients treated using a more durable therapy with a higher per unit drug cost (drug A) versus a less durable therapy with a lower per unit drug cost (drug B) in the context of a typical NHS eye hospital. Drug A can extend the interval between 2 treatments to up to 16 weeks for around 60% of patients, while drug B can extend the treatment intervals to up to 12 weeks for around 40% of patients. These treatment types were chosen to illustrate how not considering the impact of capacity constraints could lead to different decisions about resource allocation and prioritization of treatments. The characteristics of simulated patients, their treatment regimens, and intervals were informed using published literature and expert opinion to reflect real treatment options available in clinical practice. Clinic capacity was simulated by setting a limit on the number of intravitreal injection appointments available each week over a 5-year period. This number determined when demand for appointments exceeded the routine capacity available at the hospital. In this case, either the patient visit was delayed or provided as a more expensive out-of-hour service once a maximum allowed delay time was reached. After consulting experts, it was assumed that the maximum allowed delay time was 2 weeks for first appointments for nAMD, 4 weeks for nAMD follow-up appointments and DMO first appointments, and 6 weeks for DMO follow-up appointments. The model then estimated the number of patient visits, the number and duration of delays, the cost of treatment, and the loss of quality-adjusted life-years (QALYs) due to treatment delays. Technical details of the model can be found in the published study.9
Over 5 years in a resource-constrained hospital, the number of injections required for treating patients with the less durable drug B exceeded the capacity of the hospital and therefore caused treatment delays, while the number of injections required for treating patients with durable drug A did not exceed capacity. Compared with drug B, drug A resulted in the avoidance of 18,910 treatment delays, incurred about £2 million (GBP) extra costs due to higher drug acquisition cost, and avoided the loss of 106 QALYs, resulting in an incremental cost-effectiveness ratio of £19,574/QALY, which is considered good value for money according to NICE’s cost-effectiveness threshold in the United Kingdom. If the reality of capacity constraints had been ignored, decision makers may have prioritized drug B over drug A due to cost savings, leading to worse health outcomes for the population.
Discussion
This simulation study offered key insights on the inclusion of capacity constraints into economic evaluations. As expected, the introduction of a treatment option with more durable treatment effect reduces the total number of injections required, and therefore reduces the number of delayed appointments. Aside from the immediate cost savings from reduced service use, our framework allowed us to capture the benefit of avoiding delayed treatment to patients, which in our case was captured through additional QALYs accumulated over the 5-year model horizon. The cost and QALY impact were sensitive to several supply-side parameters, including the number of available clinic appointments, allowed treatment delay, and increase in demand over time, as well as some demand-side parameters, such as treatment discontinuation. As with any simulation exercise, the results are subject to uncertainty and should be interpreted with caution, although the study conclusions remained consistent when tested using sensitivity analyses.
No published studies have considered capacity constraints in the cost-effectiveness analysis of a treatment for nAMD and DMO. Some studies in other disease areas have incorporated capacity constraints into economic evaluations. For example, in oncology, pathology laboratory capacity constraint would result in a loss of net monetary benefit and reduced cost-effectiveness of a test-treat intervention.10 These studies and our study suggest that it is important to consider the impact of capacity constraints on patients and health systems when assessing the value of treatments. Ignoring the cost and QALY impact of delayed treatments can lead to inefficiencies in the allocation of scarce healthcare resources. The case study has shown that the inclusion of capacity constraints can change the conclusion of a cost-effectiveness analysis. Although less durable drug B is on the face of it “cheaper” than durable drug A in terms of the price per treatment, it was associated with considerable QALY losses arising from treatment delays. As a result, when taking capacity constraints into consideration, the more durable drug A was in fact considered cost-effective compared to drug B according to well-established thresholds such as the one adhered to by NICE in the United Kingdom.
"Economic evaluations that are able to quantify capacity constraints and incorporate them into cost-effectiveness analyses are needed in order to capture the true costs and benefits of treatments in disease areas where capacity constraints are an important consideration for healthcare decision making."
Conclusion
Incorporating capacity constraints in economic evaluations is essential to comprehensively evaluate the value of treatments. By doing so, healthcare decision makers can make informed choices that prioritize both cost-effectiveness and patient outcomes in disease areas with capacity limitations.
Capacity constraints should be considered explicitly as part of health technology assessment in therapeutic areas with limited capacity, as cheaper treatment options may not necessarily be the most cost-effective if they impose additional burden on resource-scarce systems, and the overall health benefits of more durable treatments may be undervalued. Decision makers understand the context of limited budget resources but may not fully appreciate the consequences of limitations in other healthcare resources like workforce and clinic capacity. Recognizing the “true” value of treatments in the real-world setting where health systems have limited resources is critical to ensure cost-effective and sustainable healthcare systems.
References
- NHS backlog emergency forcing people to go private or risk permanent sight loss, latest survey finds. Association of Optometrists. https://www.aop.org.uk/our-voice/mediacentre/press-releases/2023/11/23/nhs-backlog-emergency-forcing-people-togo-private-or-risk-permanent-sight-loss-latestsurvey-finds. Published November 23, 2023. Accessed June 2024.
- Gale R, Cox O, Keenan C, Chakravarthy U. Health technology assessment of new retinal treatments; the need to capture healthcare capacity issues. Eye. 2022;36(12):2236-2238 doi:10.1038/s41433-022-02149-5
- Consultant-led referral to treatment waiting times data 2022-23. NHS England. https://www.england.nhs.uk/statistics/statistical-workareas/rtt-waiting-times/rtt-data-2022-23/.Accessed June 2024.
- Workforce census 2018. The Royal College of Ophthalmologists. https://www.rcophth.ac.uk/wp-content/uploads/2020/05/RCOphth-Workforce-Census-2018.pdf. Accessed June 2024.
- Chandra S, McKibbin M, Mahmood S, et al. The Royal College of Ophthalmologists Commissioning guidelines on age macular degeneration: executive summary. Eye. 2022;36(11):2078-2083. doi:10.1038/s41433-022-02095-2
- Supporting the health and care system in improving productivity. National Institute for Health and Care. https://www.nice.org.uk/productivity. Updated February 29, 2024. Accessed June 2024.
- García-Layana A, García-Arumí J, Figueroa MS, et al. Management of wet age-related macular degeneration in Spain: challenges for treat and extend implementation in routine clinical practice. J Ophthalmol. 2019;2019:9821509. doi:10.1155/2019/9821509
- Resnikoff S, Lansingh VC, Washburn L, et al. Estimated number of ophthalmologists worldwide (International Council of Ophthalmology update): will we meet the needs? Br J Ophthalmology. 2020;104(4):588. doi:10.1136/bjophthalmol-2019-314336
- Li T, Berdunov V, Hamilton R, et al. Economic assessment in resource-constrained systems: individual-level simulation model in wet age-related macular degeneration and diabetic macular oedema. Ophthalmol Ther. 2024;13(10):2577-2597. doi: 10.1007/s40123-024-00999-8
- Wright SJ, Newman WG, Payne K. Quantifying the impact of capacity constraints in economic evaluations: an application in precision medicine. Med Decis Making. 2022;42(4):538-553. doi:10.1177/0272989x211053792