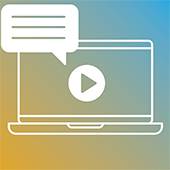
April 23, 2025
Open to all ISPOR Members and Non-members
Title: Causal AI in HEOR: Beyond Predictions to Actionable Insights
Wednesday, April 23, 2025
10:00AM EDT | 2:00PM UTC | 4:00PM CEST
Click here for time zone conversion
Register Now
Description
In recent years, the growing investment in Artificial Intelligence (AI) has raised new challenges, including the ‘black box’ problem and biases in healthcare models. While approaches like Responsible AI, Fair AI, and Explainable AI have made strides in addressing ethical concerns and promoting transparency, they each have limitations in fully accounting for causal relationships in healthcare. Causal AI, however, emphasizes the identification of cause-and-effect relationships, offering a more robust framework for analyzing health outcomes, improving decision-making, and ensuring equitable healthcare solutions.
This webinar will focus on the transformative role of causal inference in AI within the context of Health Economics and Outcomes Research (HEOR). While classical machine learning (ML) has proven effective at identifying patterns in health data, it often falls short in understanding the underlying reasons behind these patterns and how one health outcome impacts another. This limitation is particularly important in HEOR, where understanding causal relationships is crucial for evaluating the effectiveness, safety, and cost-effectiveness of healthcare interventions.
Join us for an insightful discussion on how Causal AI can improve the application of health economics and outcomes research, providing more reliable, ethical, and transparent models for assessing healthcare interventions. This webinar will feature expert insights on the potential of causal inference to reduce biases, improve policy decisions, and enhance patient outcomes, while promoting fairness and transparency in AI-driven healthcare research.
Learning Objectives
- Understand the Fundamentals of Causal Inference in AI: Participants will gain a foundational understanding of causal inference, including key concepts such as causality, correlation, and confounding factors, and how these principles differ from traditional statistical methods.
- Explore Practical Applications of Causal Inference in AI: Through research-driven examples, attendees will discover how causal inference is utilized in real-world AI systems to improve decision-making, enhance predictive accuracy, and tackle challenges related to fairness and bias in machine learning models.
- Learn Key Methodologies and Tools for Causal Inference in AI: Participants will be introduced to methodologies and tools for implementing causal inference in AI, including techniques such as causal diagrams and machine learning algorithms designed for causal analysis.
Moderator:
Omkar Ghodke, BPharm, PhD Student in Pharmaceutical Sciences, University of Mississippi School of Pharmacy, Oxford, MS, USA
Speakers:
Uwe Siebert, MD, MPH, MSc, ScD, Professor of Public Health, Medical Decision Making and Health Technology Assessment, University for Health Sciences and Technology, Medical Informatics, and Technology, Hall in Tirol, Austria
Lizheng Shi, PhD, MsPharm, MA, Neal A. and Mary Vanselow Chair in Health Management and Policy, and Director, Health Systems Analytics Research Center, Weatherhead School of Public Health and Tropical Medicine, Tulane University, New Orleans, LA, USA
Brought to you by: ISPOR Student Network Education Committee
Please note: On the day of the scheduled webinar, the first 1000 registered participants will be accepted into the webinar. For those who are unable to attend, or would like to review the webinar at a later date, the full-length webinar recording will be made available at the ISPOR Educational Webinar Series webpage approximately 2 days after the scheduled Webinar.