The Role of External Control Arms in Drug Development and Considerations for Success
Alexandra Z. Sosinsky, ScM, Craig S. Parzynski, MS, Deborah Casso, MPH, Genesis Research Group, Hoboken, NJ, USA
Introduction
External control arm (ECA) studies are typically pursued to meet evidentiary standards of regulatory and/or health technology assessment (HTA) bodies and developed to complement single-arm trials. While randomized controlled trials (RCTs) are the gold standard for decision making, ECA studies can be a valuable tool under appropriate circumstances. Strong justification for the use of a single arm trial and corresponding ECA study is needed for submissions to regulatory and HTA bodies. Circumstances that may necessitate a single-arm trial rather than a RCT include debilitating or life-threatening diseases with no available treatment, enrollment challenges, or where clinical equipoise does not exist. In an ECA study, patients from an external data source are carefully selected to represent the clinical trial target patient population, and then the ECA patients are indirectly compared to patients in the single-arm trial. ECA studies have been used to demonstrate the efficacy of experimental therapies, most commonly for some types of cancer and rare diseases.1
"There has been a growing interest in the use of ECA studies and their potential impact on drug development."
Typical external data sources used for ECAs include prior clinical trial data or real-world data (RWD) from administrative claims databases, electronic health records, patient registries, or medical chart abstraction from which individual patient data can be derived.2 There has been a growing interest in the use of ECA studies and their potential impact on drug development, including the possibility of:
- Expediting drug development and therefore, the availability of novel treatments to patients
- Cost savings compared to a traditional RCT
- Providing stakeholders with valuable information on effectiveness
To fulfill the needs of stakeholders, including patients and providers, ECA studies must be carefully planned, designed, executed, and reported in a way that reflects the complexity of the clinical condition and provides the most appropriate results.
Methodologic Considerations
While an ECA can be designed as a benchmark study without a formal comparison to contextualize single-arm trial data, a formal comparative effectiveness study would require
more stringent design considerations. The methodologic considerations described below focus on comparative ECA studies.
Broadly speaking, the conduct of an ECA study can be broken down into 4 phases: planning, design, analysis, and reporting (Figure 1). Below, we outline important methodologic considerations by phase.
Figure 1. The 4 phases of an external control arm study
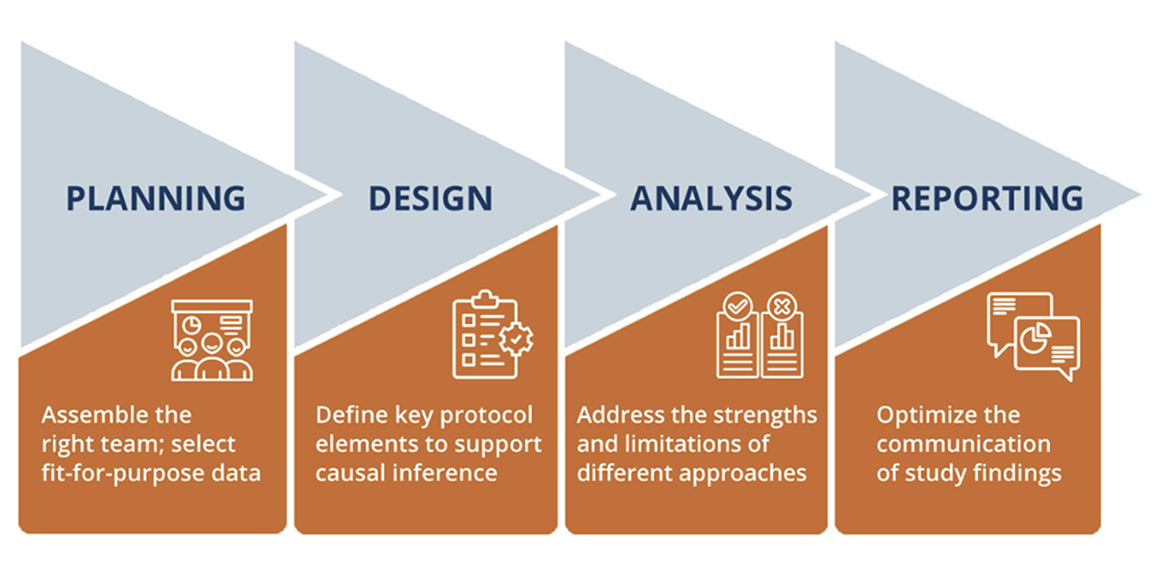
Planning: Begin planning early for an ECA Study
The planning stage should ideally begin in parallel to early development and clinical trial design. An ECA study may still be desired in the absence of early planning (eg, the design phase overlapping with a trial read-out) although stakeholders may challenge whether the chosen data sources and specific analyses were conducted to produce a certain finding.
In the planning stage, assembly of the right team and selection of a fit-for-purpose data source are crucial for success. A successful ECA will require input from epidemiologists, biostatisticians, clinicians, and regulatory experts. ECA studies are complex, causal inference studies that require cross-functional stakeholders. A qualified team will appropriately shape the study in the early stages, proactively identify potential criticisms, and validate the study design and data source choices, which is invaluable for efficiency.
The optimal ECA source must be identified by considering the specific research question and selected through a systematic and rigorous fit-for-purpose evaluation (eg, by following published evaluation frameworks).3,4 Key considerations for data source selection include the ability to apply adapted trial inclusion and exclusion criteria, expected sample size, comparability of the endpoints, validity of the endpoints and other key variables, length of follow-up, availability of prognostic variables, and a thorough understanding of missing data. Even the most rigorous study design and statistical analysis methods cannot overcome data that are not fit-for-purpose.
Design: Prespecification of the ECA study design to support causal inference
Given the lack of randomization and blinding in a single-arm trial, a combination of the target trial and International Conference on Harmonization E9(R1) estimand frameworks5 can be used to define key protocol elements of the hypothetical RCT that would answer the causal question of interest (the “target trial”) and describe how each item can be emulated given the available data.6,7 Approaching the ECA study design this way helps provide clarity on the causal quantity being estimated and address common threats to internal validity.
Common issues encountered in the design of ECA studies include confounding, selection bias, immortal time bias, and a lack of comparable outcomes (Table). These issues should be addressed to the extent possible in the study design and analysis, which need to be prespecified in a study protocol and statistical analysis plan in advance of data analysis. The HARmonized Protocol Template to Enhance Reproducibility (HARPER) template, created by a joint task force between the International Society for Pharmacoepidemiology (ISPE) and ISPOR—The Professional Society for Health Economics and Outcomes Research, provides a helpful framework for communicating study designs in a clear, transparent, and reproduceable manner.8 It is recommended that the study protocol be registered on a site like Clinicaltrials.gov or the HMA-EMA Catalogues of Real-World Data Sources and Studies in advance of any trial results readouts so that decision makers (eg, regulators, payers) can be confident the trial results had no influence on the chosen methods for the ECA study.3,9 Finally, engaging regulators early to reach alignment on the anticipated study will help ensure successful submissions.
Table. Common Biases Encountered in External Control Arm Studies
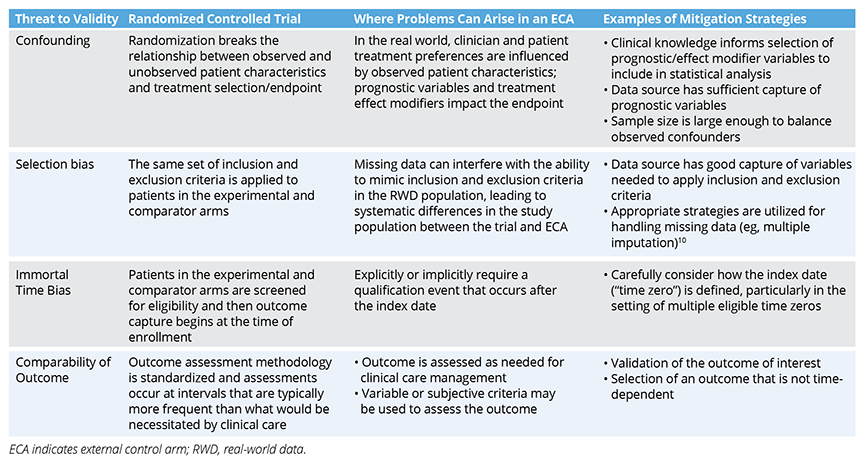
Analysis: The strengths and limitations of various statistical approaches should be considered to fit the specific ECA
The methods for analyzing data from externally controlled trials will differ depending upon the research question, choice of data source, and other study-specific factors. Propensity score-based methods are most commonly used, although a variety of causal inference methods exist to address confounding and approximate randomization, such as targeted maximum likelihood estimation, marginal structural models, and G-methods. Assumptions for the chosen analytic plan should be described and those assumptions should be examined through sensitivity analyses and model diagnostics.11 Specifically, since causal inference methods only account for measured confounding, sensitivity analyses to assess the potential for unmeasured confounding are critical to interpreting results and understanding their validity.
"ECA studies can expedite the development of novel treatments, potentially reduce research costs, and offer needed insights into comparative effectiveness, all of which ultimately benefits patients in need of innovative therapies."
Other analysis considerations include:
- Missing data: Missing data are common in most data sources used for ECAs. Missingness patterns and mechanisms should be assessed and appropriate methods should be incorporated.
- Intercurrent events: These are events that occur after treatment initiation that affect either the interpretation or existence of measurements related to the endpoint. Intercurrent events should be discussed in the design phase and specific methods to handle intercurrent events should be incorporated into the analysis phase.
- Multiple index dates: Research has shown that including all eligible index dates for patients in the ECA leads to the least bias in treatment effect estimates.12,13 Methods used must account for a patient being included multiple times (eg, a patient meeting eligibility criteria at the initiation of multiple lines of therapy).
- Time-varying confounding: Depending on the treatment effect of interest, important prognostic factors or effect modifiers that change after enrollment may need to be controlled for.
Reporting: Communication of ECA findings to stakeholders and the broader public
Dissemination of the ECA study results to stakeholders and the broader public is an essential step to ensuring that the study can impact decision making and clinical care, as appropriate. Available guidelines, such as the STrengthening the Reporting of OBservational studies in Epidemiology (STROBE) and ISPE’s Guidelines for Good Pharmacoepidemiology Practices,14,15 provide helpful guidance for the reporting of observational study findings, including ECA studies. We highlight a few key elements:
- Aim to publish in a peer-reviewed journal
- Be transparent with respect to results reporting (eg, differentiate prespecified versus ad hoc analyses and avoid selective reporting of results from the study16)
- Disclose all conflicts of interest and sources of study funding
- Discuss study limitations and uncertainty
Takeaways and Future Outlook
ECA studies play a critical role in contextualizing the information learned through single-arm clinical trials. At their best, ECA studies can expedite the development of novel treatments, potentially reduce research costs, and offer needed insights into comparative effectiveness, all of which ultimately benefits patients in need of innovative therapies. Existing methods and data sources often fall short of addressing stakeholder concerns,17 and we recommend:
- Earlier planning and engagement with regulators—in conjunction with clinical trial planning—can increase validity and impact of the ECA study.
- Strong partnerships with data providers to identify fit-for-purpose data and increase the reliability and validity of endpoints in RWD to fulfill regulatory, and increasingly HTA body, expectations for outcome validation.
- Rigorous methods to ensure valid conclusions, including sensitivity analyses to understand unmeasured confounding.
It is not expected that ECA studies would ever replace RCTs. However, for therapies and conditions where an ECA study is justified, with good planning, design, implementation, and reporting, ECA studies can help fill a knowledge gap and enhance treatment options for patients.
References
1. Patel D, Grimson F, Mihaylova E, et al. Use of external comparators for health technology assessment submissions based on single-arm trials. Value Health. 2021;24(8):1118-1125. doi:10.1016/j.jval.2021.01.015
2. Seeger JD, Davis KJ, Iannacone MR, et al. Methods for external control groups for single arm trials or long-term uncontrolled extensions to randomized clinical trials. Pharmacoepidemiol Drug Saf. 2020;29(11):1382-1392. doi:10.1002/pds.5141
3. US Food and Drug Administration (FDA). Considerations for the use of real-world data and real-world evidence to support regulatory decision-making for drug and biological products: Guidance for industry. 2023. Accessed November 20, 2023. https://www.fda.gov/regulatory-information/search-fda-guidance-documents/considerations-use-real-world-data-and-real-world-evidence-support-regulatory-decision-making-drug
4. European Medicines Agency (EMA). Data Quality Framework for EU medicines regulation. 2023. Accessed November 20, 2023. https://www.ema.europa.eu/en/documents/regulatory-procedural-guideline/data-quality-framework-eu-medicines-regulation_en.pdf
5. Hampson LV, Chu J, Zia A, et al. Combining the target trial and estimand frameworks to define the causal estimand: an application using real-world data to contextualize a single-arm trial. Stat Biopharm Res. 2023:1-10. doi:10.1080/19466315.2023.2190931
6. Hernán MA, Robins JM. Using big data to emulate a target trial when a randomized trial is not available. Am J Epidemiol. 2016;183(8):758-764. doi:10.1093/aje/kwv254
7. International Council for Harmonisation (ICH). E9 (R1) Addendum on estimands and sensitivity analysis in clinical trials to the guideline on statistical principles for clinical trials. 2019. Accessed November 20, 2023.https://database.ich.org/sites/default/files/E9-R1_Step4_Guideline_2019_1203.pdf
8. Wang SV, Pottegård A, Crown W, et al. HARmonized Protocol Template to Enhance Reproducibility of hypothesis evaluating real-world evidence studies on treatment effects: A good practices report of a joint ISPE/ISPOR task force. Value Health. 2022;25(10):1663-1672. doi:10.1016/j.jval.2022.09.001
9. National Institute for Health and Care Excellence (NICE). NICE real-world evidence framework. 2022. Accessed November 20, 2023. https://www.nice.org.uk/corporate/ecd9/chapter/overview
10. Austin PC, Giardiello D, van Buuren S. Impute-then-exclude versus exclude-then-impute: Lessons when imputing a variable used both in cohort creation and as an independent variable in the analysis model.
Stat Med. 2023;42(10):1525-1541. doi:10.1002/sim.9685
11. US Food and Drug Administration (FDA). Considerations for the design and conduct of externally controlled trials for drug and biological products. 2023. Accessed November 20, 2023. https://www.fda.gov/regulatory-information/search-fda-guidance-documents/considerations-design-and-conduct-externally-controlled-trials-drug-and-biological-products
12. Backenroth D. How to choose a time zero for patients in external control arms. Pharm Statistics. 2021;20(4):783-792. doi:10.1002/pst.2107
13. Hatswell AJ, Deighton K, Snider JT, Brookhart MA, Faghmous I, Patel AR. Approaches to selecting “time zero” in external control arms with multiple potential entry points: a simulation study of 8 approaches. Med Decis Making. 2022;42(7):893-905. doi:10.1177/0272989X221096070
14. Public Policy Committee International Society for Pharmacoepidemiology. Guidelines for good pharmacoepidemiology practice (GPP). Pharmacoepidemiol Drug Saf. 2016;25(1):2-10. doi:10.1002/pds.3891
15. Von Elm E, Altman DG, Egger M, Pocock SJ, Gøtzsche PC, Vandenbroucke JP. The Strengthening the Reporting of Observational Studies in Epidemiology (STROBE) statement: guidelines for reporting observational studies. Lancet. 2007;370(9596):1453-1457. doi:10.1016/S0140-6736(07)61602-X
16. Orsini LS, Berger M, Crown W, et al. Improving transparency to build trust in real-world secondary data studies for hypothesis testing—why, what, and how: recommendations and a road map from the real-world evidence transparency initiative. Value Health. 2020;23(9):1128-1136. doi:10.1016/j.jval.2020.04.002
17. Jaksa A, Louder A, Maksymiuk C, et al. A comparison of 7 oncology external control arm case studies: critiques from regulatory and health technology assessment agencies. Value Health. 2022;25(12):1967-1976. doi:10.1016/j.jval.2022.05.016
Acknowledgments: The authors thank Kristen Downs, MSPH and Richard Chumbley, MA of Genesis Research Group, Hoboken, NJ, USA, for providing editorial support.