Multilevel Network Meta-Regression
Section Editor: Koen Degeling, PhD
We are introducing a new section called “Methods Explained” in this issue of Value & Outcomes Spotlight, which will provide a high-level overview of a specific method based on the Section Editor’s conversations with experts. Inspired by a keen interest in methods, the section aims to provide readers with an understanding of whether and when certain methods may be applicable to their work and offer a starting point for further reading. The column will cover the intent of the method, how it compares to alternatives, to what extent it has been implemented, and what challenges remain in its use and application. This first edition will cover multilevel network meta-regression and future editions may discuss methods like distributional cost-effectiveness analysis and best-worst scaling for stated preference research. Suggestions on topics to be covered and experts to be interviewed are welcome and can be shared with the Value & Outcomes Spotlight Editorial Office.
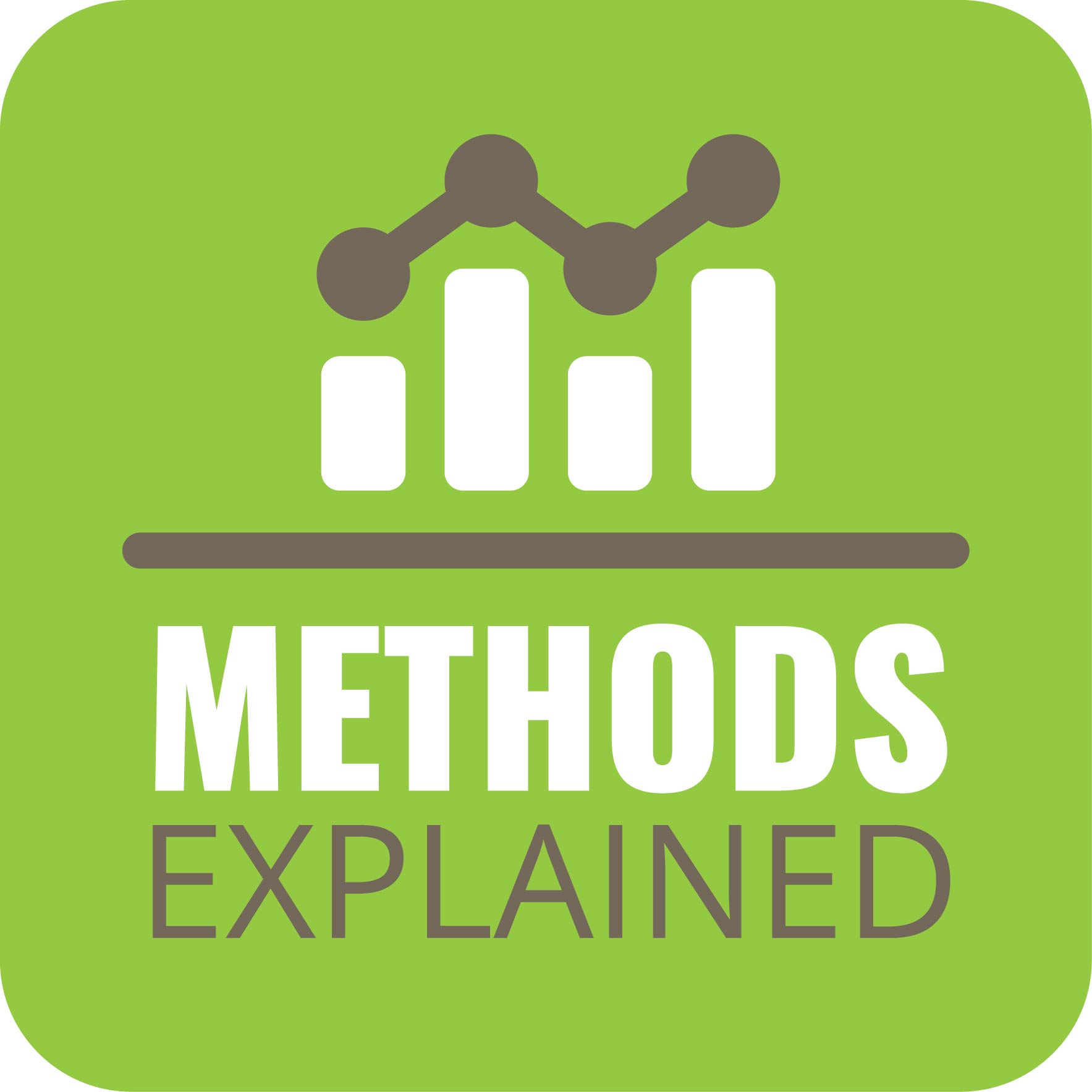
For this edition of “Methods Explained,” I have spoken to 2 experts who play an important role in the adoption of multilevel network meta-regression (ML-NMR) in the field of health economics and outcomes research (HEOR). Jeroen Jansen, PhD is an Associate Professor at the Department of Clinical Pharmacy of the University of California, San Francisco and Chief Scientist, HEOR at the Precision Medicine Group. Drawing on methods used in ecology, Jeroen first introduced a precursor to ML-NMR back in 2012.1 David Phillippo, PhD is a Research Fellow in Evidence Synthesis at the Bristol Medical School of the University of Bristol. David generalized Jeroen’s ideas into the ML-NMR framework and is the author of the multinma R package, which is playing an important role in the broader adoption of ML-NMR.
What is the objective of the method?
As an evidence synthesis method, ML-NMR aims to combine evidence from multiple sources to estimate relative treatment effects for decision making or research purposes in general. In many circumstances, multiple randomized controlled trials have been performed to estimate relative treatment effects and the evidence from these trials is to be combined. In doing so, there may be differences in population characteristics between studies that can impact the measured treatment effect. ML-NMR combines evidence from a collection of studies and accounts for differences in population characteristics to provide estimates of treatment effects between all treatments of interest. This aim of combining evidence from a collection of studies is conceptually the same as that of network meta-analysis, a well-known and widely used method in HEOR.
What makes it different from similar methods?
Standard network meta-analysis relies on aggregate data, such as estimates reported in scientific articles, and does not account for potential differences between the characteristics of the participants in clinical studies. This may result in biased estimates if such differences concern characteristics that impact the treatment effect, so-called effect modifiers. The “gold standard” approach is a network meta-regression combining individual-level data from every study, adjusting for differences in effect modifiers between study populations to provide unbiased estimates of treatment effects. However, it is rare for individual-participant data to be available for all studies and, often, a mixture of aggregate and individual-level data needs to be combined to consider all the evidence available. ML-NMR coherently combines both individual- and aggregate-level data, accounting for this common scenario where different levels of data are available from different studies (hence, “multilevel”). One could say that ML-NMR encompasses both aggregate-data network meta-analysis and individual-participant data NMR: it reduces to standard network meta-analysis when only aggregate data are used, and to the “gold standard” NMR if individual-level data are available for all studies.
For those that are familiar with evidence synthesis methods, this may sound like matching-adjusted indirect comparisons and simulated treatment comparisons. However, when using matching-adjusted indirect comparisons or simulated treatment comparisons, treatment effects can only be estimated for the study population as observed in the aggregate data. In other words, the target population in your research question has to be the same as the aggregate data study population for these methods to be useful. ML-NMR does not have this limitation. This is a very important benefit, because treatment decisions or conclusions may be different between populations with differences in their distributions of effect modifiers. Furthermore, matching-adjusted indirect comparisons and simulated treatment comparisons can only compare individual-level data studies to aggregate data from a single trial, whereas ML-NMR can combine individual-participant and aggregate data from any number of studies.
In summary, ML-NMR is different from other methods given that it is able to combine both aggregate and individual-level data and is able to provide effectiveness estimates for a target population that is relevant to the research question and does not have to match that of the aggregate data used.
What are the steps involved in applying the method?
On a high-level, the steps for performing a ML-NMR are the same as those for a network meta-analysis: defining the research question, deciding on the model to use, gathering the data, running the analysis, and assessing the impact of key assumptions. However, certain aspects must be more explicitly and transparently defined, mainly the selection of effect modifiers and the definition of the target population of interest.
Running an ML-NMR can be considered more complex compared to running a standard network meta-analysis, which can be easily done in various software. This has likely been a barrier to the broader adoption of ML-NMR. With the publication of Phillippo’s multinma R package, a relatively user-friendly approach is offered for running ML-NMR analyses in a software that is commonly used for other evidence synthesis and data analysis tasks.
To what extent has the method been used in practice?
Although ML-NMR is recommended by the National Institute for Health and Care Excellence (NICE) Decision Support Unit in the United Kingdom as a preferred method for population-adjusted indirect comparisons,2 there have only been a few applied examples across disease areas. For example, it was used in NICE TA912 that evaluated cipaglucosidase alfa with miglustat for treating late-onset Pompe disease, where it was well-received by the Evidence Assessment Group.3 Given the important advantages it has over other methods and with the introduction of the multinma R package, the number of applications is expected to increase over the coming years.
What is next for the method?
Given that other methods like matching-adjusted indirect comparisons are better known and well-understood, broader awareness of ML-NMR and an improved understanding will be important to ensure the HEOR community can leverage the advantages it provides.
Different extensions of the method may further increase its applicability. For example, Jansen and Phillippo particularly see value in facilitating parameterization of full multistate models, which are a particular type of decision-analytic model that can be used to evaluate the health and economic outcomes of different healthcare interventions. Another opportunity lays in the incorporation of single-arm trials and disconnected evidence networks, which is highly relevant given the increased use of single-arm studies in particular disease areas.
What are some key references for further reading?
Those who would like to learn more about ML-NMR may have interest in reading a “walkthrough” paper based on a case study in plaque psoriasis4 or a more foundational paper.5
Did you enjoy reading this article? Or do you have any suggestions for methods to be covered in future editions? Please share your feedback with the Value & Outcomes Spotlight Editorial Office.
References
1: Jansen JP. Network meta-analysis of individual and aggregate level data. Res Synth Methods. 2012;3(2):177-90.
2: Welton NJ, Phillippo DM, Owen R, et al. DSU Report. CHTE2020 Sources and Synthesis of Evidence; Update to Evidence Synthesis Methods. March 2020. Accessed July 22, 2024. https://www.sheffield.ac.uk/nice-dsu/methods-development/chte2020-sources-and-synthesis-evidence
3: Cipaglucosidase alfa with miglustat for treating late-onset Pompe disease. NICE technology appraisal guidance 912. 2015. Accessed July 22, 2024. https://www.nice.org.uk/guidance/ta912
4: Phillippo DM, Dias S, Ades AE, et al. Validating the assumptions of population adjustment: application of multilevel network meta-regression to a network of treatments for plaque psoriasis. Med Decis Making. 2023;43(1):53-67.
5: Phillippo DM, Dias S, Ades AE, et al. Multilevel network meta-regression for population-adjusted treatment comparisons. J R Stat Soc Ser A Stat Soc. 2020;183(3):1189-1210.