Discrete Event Simulation
Section Editor: Koen Degeling, PhD
In this edition of Methods Explained we are covering discrete event simulation (DES) based on a conversation with Hendrik (Erik) Koffijberg, PhD. He is professor of Technology Assessment of Digital Health Innovations and chair of the section Health Technology & Services Research at the University of Twente in The Netherlands, where he teaches several courses on health economic modeling. He is also a member of the scientific advisory board of the National Healthcare Institute. Together with other experts, Erik and I have published several methodological and practical papers on DES, and we continue to teach postgraduate courses and short courses on the topic.
What is DES and what can it be used for?
DES is a simulation technique that originates from operations research. It dates back to the 1950s and has been used extensively to investigate and optimize logistic, manufacturing, and operational processes that are characterized by scarce resources and queues.
In line with its origin, the first applications of DES in healthcare focused on optimizing care processes on an operational level, such as in hospitals. Examples include optimizing emergency department layout and staffing, as well as operating room scheduling, which are settings characterized by limited resources and variable demand.
DES has also proven valuable as a decision-analytic modeling technique within the field of health economics and outcomes research (HEOR). Like decision trees, Markov models and partitioned-survival models, it can be used to perform model-based economic analyses, such as cost-effectiveness analyses. It is also well suited to model the (re)design or optimization of health services and support implementation decisions.
How does DES work and what makes it different from similar methods?
To understand how DES works, it is useful to compare it to other well-known and commonly used cohort-level modeling methods, such as partitioned-survival modeling and Markov modeling (ie, cohort-level state-transition modeling), and to other individual-level modeling methods (ie, microsimulation), such as individual-level state-transition modeling.
DES is an individual-level modeling method like individual-level state-transition modeling, which implies that it simulates hypothetical individuals or “entities,” each with their own characteristics and history. This is different from cohort-level methods, such as Markov modeling and partitioned-survival modeling, which model an average cohort by keeping track of proportions of the cohort assigned to certain health states over time. By simulating individuals, DES is able to account for differences in events and outcomes between individuals that can be explained (ie, heterogeneity) and that arise due to random chance (ie, stochastic or first-order uncertainty).
Something that really sets DES apart is its handling of time. Most state-transition models are discrete-time methods, which means that they deal with time in fixed (discrete) steps by repeatedly applying state transitions based on probabilities. This is illustrated in the hypothetical example in Figure 1, where transitions to different (or the same) health states are applied repeatedly. On the other hand, DES is a continuous-time method and it jumps from event to event, which can occur at any point in time. The impact of this difference is that DES is more efficient and potentially more accurate compared to discrete-time methods. Furthermore, this makes it straightforward to implement parametric and empirical time-to-event distributions in a DES, with parametric distributions being commonly used in HEOR to extrapolate observed survival data.
Figure 1. Illustration of the model structure and handling of time for the commonly used 3-state oncology model for: (a) a discrete-time state-transition model (eg, Markov model) and (b) a discrete event simulation model.
Another difference between DES and state-transition models is that the latter are typically structured based on health states, whereas DES models are often specified based on more granular events or processes where the health state is defined as a characteristic of the simulated individuals. Figure 2 presents a model structure that resembles the same clinical pathway as Figure 1 but is structured based on more granular events that can be mapped directly to the impact of those events, such as the cost and impact on quality of life of starting a new treatment. This decoupling of the model structure and health states may allow for a more straightforward and realistic translation of clinical pathways into model structures. For example, this makes it possible to model diagnostic tests without those necessarily impacting the health state of the individual. It also allows more detailed and transparent definition of costs and health effects in the model, by linking these to the granular events in the model structure.
Figure 2. Illustration of how the commonly used three-state oncology model of Figure 1 could also be implemented as a care process based on more detailed events and with health states modeled as characteristic for each of the simulated individuals.
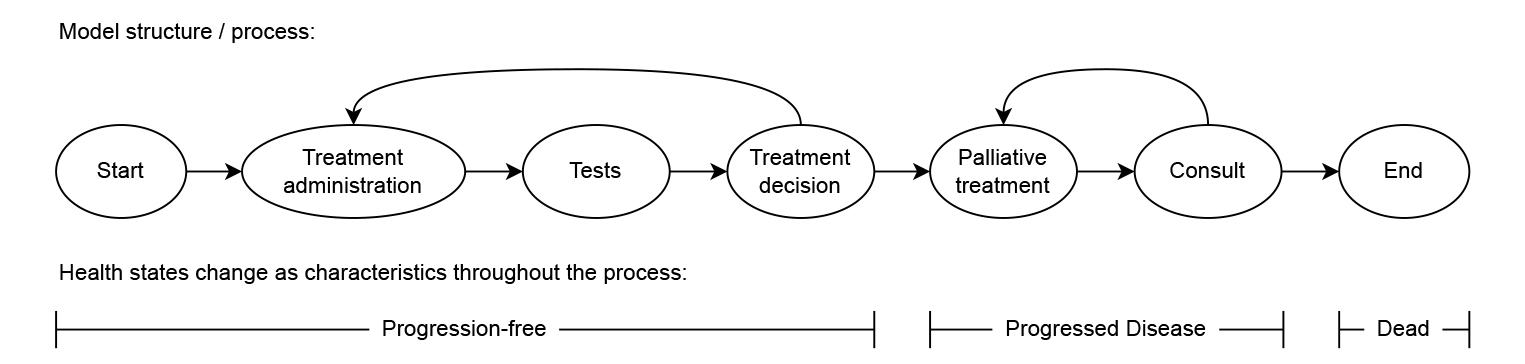
Finally, its ability to readily model resources and capacity constraints, and how simulated individuals interact with each other through those resources, are unique to DES. Examples of resources in a healthcare setting include machinery, such as certain hospital or laboratory equipment, but certainly also the time of healthcare providers. Queues and waiting times, and their impact on relevant outcomes, can easily be simulated by specifying required and available resources. Although interaction between entities of the same type (eg, patients) can theoretically be modeled using DES, this is highly complex due to its continuous handling of time and, hence, this is typically done using agent-based models (a discrete-time individual-level modeling method).
Overall, compared to more traditional modeling techniques used in HEOR, DES offers increased flexibility to represent complex clinical pathways without simplifying assumptions and facilitates consideration of resources and capacity constraints. Therefore, DES would be the preferred technique when explicitly modeling resources, whereas for typical health economic models, the added value of DES increases with increasing complexity of the modeled pathway and larger amounts of information being available to populate the model.
What are the steps in using DES and what software is available?
Fundamentally, there are no differences in the steps involved in applying DES compared with individual-level state-transition modeling. What makes these microsimulation methods different from the cohort-level techniques is the simulation of the population of hypothetical individuals with their (correlated) characteristics and the need to assess whether sufficient individuals are simulated to obtain stable outcome estimates.
In terms of software, DES has traditionally been available through proprietary software, such as Simul8, AnyLogic, Arena, and TreeAge. Like state-transition models, relatively simple DES models that do not consider resources can be implemented in Excel, but such applications seem to be rare. As an alternative, freely available code-based environments like R and Python have been used increasingly for DES, with R being most prominently used within HEOR. Although proprietary software generally provides convenient user interfaces, they can be costly and may not necessarily be as transparent, flexible, and reusable as code-based alternatives. With initiatives like the R for Health Technology Assessment (HTA) consortium, the use of code-based environments to implement DES models within HEOR may increase further.
What is the current level of adoption of DES in HEOR?
DES has been used widely in research and organizations across various disciplines, including healthcare organizations, where it has been used to inform decisions on the organization of care on national, regional, and local levels. It is increasingly used in HEOR and there are plenty of DES applications in academic HEOR projects. Although it is accepted as a modeling technique by most HTA agencies, use in reimbursement submissions has been relatively rare.
The use of DES in HTA submissions may increase over the coming years as clinical pathways continue to become more complex. There may also be more applications due to an increasing interest in considering workforce capacity and environmental sustainability as part of HTA (eg, at the National Healthcare Institute in The Netherlands). Increased use of advanced evidence synthesis methods to analyze and combine information from various sources, such as multilevel network-meta regression that was covered in a previous edition of Methods Explained, may also contribute to the further adoption of DES. Individual-level techniques like DES can fully utilize the output of such analyses, whereas cohort-level techniques require such outputs be aggregated to the average cohort being modeled, resulting in a loss of information.
What are the remaining challenges in the uptake of DES?
There are several perceived challenges regarding the use of DES. The computational burden of individual-level modeling techniques in general was a barrier to their use previously, but nowadays these models can be easily run on a standard laptop. Potentially the biggest perceived challenge is that individual-level models, or DES specifically, need more data compared to cohort-level state-transition models. This is not true. If there is scant but sufficient evidence to develop a Markov model, the same evidence can be used to develop a DES model. The added value of DES in that scenario may be limited, although it may still facilitate a straightforward integration of the evidence and more accurate representation of clinical practice, especially for complex pathways. More detailed evidence becoming available at a later stage would also be more easily implemented in a DES model, through more granular events.
As most HEOR professionals are trained in using state-transition models, the steep learning curve is a real barrier to using DES. For example, it is typically required to write at least some code to implement a DES. The most challenging aspect, however, turns out to be the model conceptualization. Because DES offers a lot of flexibility, there are often multiple ways to translate a clinical pathway full of events of varying importance into a model structure. With increasing familiarity through methodological guidance, examples, tutorials and (short) courses, as well as a new generation of modelers being trained in code-based software like R, the barriers to leveraging the power of DES will likely continue to decrease.
What are some key references for further reading?
A good starting point for reading more about DES in the context of HEOR is the ISPOR-SMDM Task Force report.1 However, given that substantial progress has been made in adapting the DES methodology for HEOR since its publication, this report from 2012 is not fully up to date anymore. Throughout 2025, a tutorial and book chapter on the implementation of health economic DES models in R are expected, but these have not been published yet.
In terms of examples, a review by Vázquez-Serrano et al presents various operational research studies leveraging DES in healthcare.2 With regard to HEOR, one of my own publications showcases the value of DES for modeling the complex treatment pathway of prostate cancer based on only aggregate data from literature.3 The work by Blommestein et al illustrates how real-world data can be combined in a DES model with effect estimates from network meta-analyses to model treatment sequences in multiple myeloma.4
References
- Karnon J, Stahl J, Brennan A, Caro JJ, Mar J, Möller J. ISPOR-SMDM Modeling Good Research Practices Task Force. Modeling using discrete event simulation: a report of the ISPOR-SMDM Modeling Good Research Practices Task Force--4. Value Health. 2012;15(6):821-827.
- Vázquez-Serrano JI, Peimbert-García RE, Cárdenas-Barrón LE. Discrete-event simulation modeling in healthcare: a comprehensive review. Int J Environ Res Public Health. 2021;18(22):12262.
- Degeling K, Corcoran NM, Pereira-Salgado A, Hamid AA, Siva S, IJzerman MJ. Lifetime health and economic outcomes of active surveillance, radical prostatectomy, and radiotherapy for favorable-risk localized prostate cancer. Value Health. 2021;24(12):1737-1745.
- Blommestein HM, Franken MG, van Beurden-Tan CHY, et al. Cost-effectiveness of novel treatment sequences for transplant-ineligible patients with multiple myeloma. JAMA Netw Open. 2021;4(3):e213497.
We welcome feedback and suggestions for topics or experts in the field for future issues. Please contact the Value & Outcomes Spotlight editorial office at voseditor@ispor.org with your ideas.